Learn from past operation result information to appropriately estimate future operation times
FLEXSCHE AutoCalibrator
FLEXSCHE AutoCalibrator
"Creating a highly accurate plan that aligns with reality" is one of the major challenges in production scheduling. For this, it is a necessary condition to be able to appropriately estimate operation times during planning.
However, in a manufacturing environment that changes daily due to a wide variety of products and shortened product life cycles, it is difficult to fully grasp these factors.
FLEXSCHE AutoCalibrator contributes to the creation of highly accurate and reasonable plans by learning from the operation results of manufacturing and estimating appropriate operation times during planning.
Construct a machine learning model from operation results to estimate operation times
The operation time required during manufacturing generally varies due to various factors.
For example, the changeover time required when switching the product being manufactured can vary due to factors such as material, color, width, thickness, the jigs used, and the machines used.
To accurately estimate these, it is essential to conduct interviews with the field. Continuously doing this to match the ever-changing manufacturing environment clearly requires a lot of effort.
FLEXSCHE AutoCalibrator can estimate operation times without master maintenance by learning operation result information from the field and constructing a machine learning model.
By providing the various conditions and the operation time taken when the operation was actually performed to the machine learning model, it learns how the operation time changes depending on the conditions.
Once the learning is complete and the machine learning model is constructed, you can provide the various conditions for the upcoming operations during planning to appropriately estimate the operation times.
Tune capacity values based on estimation
If the operation time can be calculated with a simple model, such as time being proportional to the quantity produced or varying by person but not by quantity, you can use the data tuner function.
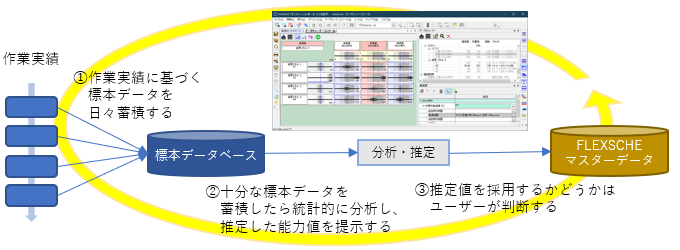
The data tuner function accumulates sample data of operation times based on operation results daily and presents estimated values calculated from statistical processing results.
After the data tuner operation starts, a sufficient number of sample data will accumulate over time, allowing for analysis. The relationship between the current capacity values, the newly estimated capacity values, and the distribution of sample values is visualized, and the "recommendation level" of the new capacity values is also presented, allowing users to quickly and intuitively update capacity values.
Data Tuner Console (left) and Data Tuner Panel (right)
(If capacity is parameterized by skills or numerical specifications, it is analyzed in multiple dimensions)
It is common to assign a common capacity value to multiple machines considered to have the same performance, but significant capacity differences are often found when actually used. When the data tuner detects this difference, it visualizes and presents a splitting proposal, allowing you to easily split the capacity definition with simple operations.
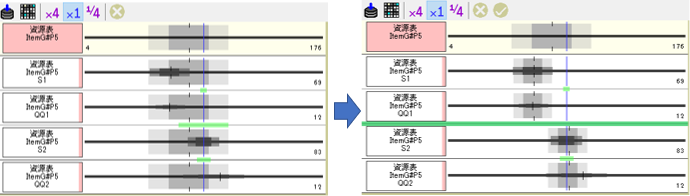
Before (left) and after (right) splitting capacity value settings
Confirm the coexistence of resources with different capacities, and split with just a double-click operation
By utilizing these mechanisms, you can initially assign rough capacity values and gradually subdivide and detail them, making it easier to build a production scheduling system.
In conjunction with FLEXSCHE CarryOut
Statistically reasonable plans form the foundation for appropriate plan execution, but they are not sufficient on their own.
This is because the aforementioned "fluctuations" hinder manufacturing according to the plan. It is important to accept and adapt to these "fluctuations" while executing the plan, and the mechanism for this is FLEXSCHE CarryOut. By appropriately operating FLEXSCHE CarryOut, you can naturally obtain accurate result information, creating a complementary relationship that is beneficial in two ways.